Measuring eCommerce offer incrementality with control groups
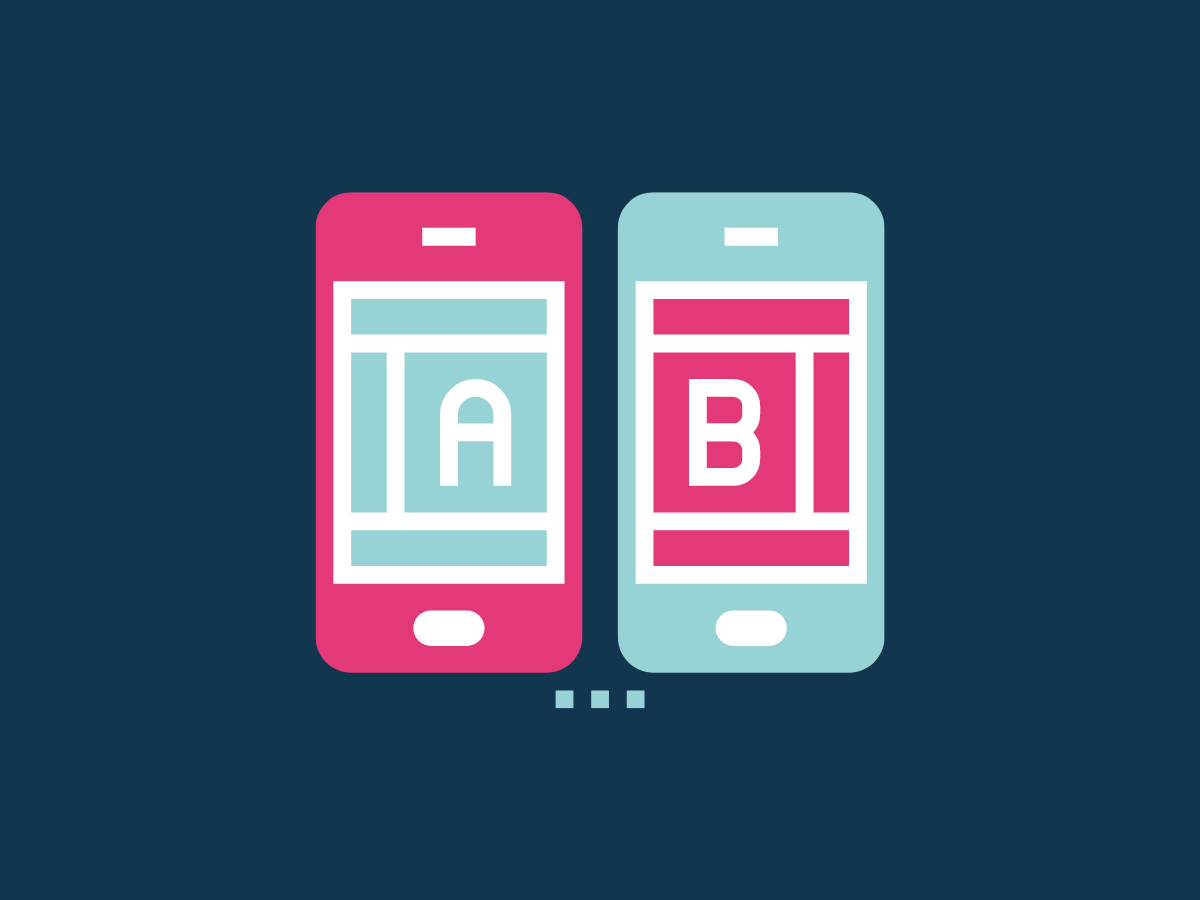
Incrementality. Now, there's a word.
It refers to measuring additional business outcomes directly attributable to a marketing campaign or activity. In our case, this means understanding the impact of a particular promotional offer on sales and revenue.
Traditionally, marketers have relied on metrics such as click-through rates (CTR) and return on ad spend (ROAS) to gauge the success of their campaigns. However, these metrics only provide a partial view of the overall impact and do not consider external factors or the incremental lift caused by the campaign.
RevLifter uses control group reporting to measure incrementality and ensure offers and campaigns drive better results.
Why not just use attribution?
Attribution reporting faces a host of challenges that can undermine its effectiveness in measuring the true performance of marketing campaigns:
- Overemphasis on the last-click
Traditional models often prioritize the last interaction before conversion, underestimating the value of everything else in the decision-making process. - Ignoring offline channels
Many attribution models fail to incorporate the influence of offline marketing activities, such as in-store promotions, on online sales and engagements. - Cookie reliance and privacy concerns
The growing emphasis on user privacy and the gradual phasing out of third-party cookies challenge the effectiveness of attribution tracking. - Cross-device tracking
Consumers frequently switch between devices during their purchase journeys. Many attribution models struggle to link interactions across mobiles, tablets, and desktops, leading to gaps in the data. - Simplification of behavior
Attribution models often oversimplify the complexity of consumer behaviors, not accounting for external factors such as word-of-mouth, market trends, or economic changes.
Addressing these challenges requires more sophisticated and holistic approaches to understanding marketing effectiveness.
The benefits of accurate incrementality measurement
Having a robust understanding of incrementality can bring several advantages for eCommerce brands. It allows them to:
- Accurately evaluate the effectiveness of different marketing campaigns and offers
- Identify the most profitable channels and optimize budget allocation
- Determine the optimal timing for running promotions and offers
- Gain insights into customer behavior and preferences
Is incrementality measurement difficult?
Measuring incrementality accurately can pose some challenges that can complicate the assessment of a campaign's true effectiveness:
- Establishing a control group
Identifying and maintaining a control group representing the target audience can be difficult. This group must not be exposed to the campaign to measure the incremental effect accurately. - Attribution
In multi-channel marketing, attributing sales to a single campaign can be complex. Consumers may interact with multiple touchpoints before making a purchase, making it hard to determine which interaction was truly incremental. - External influences
Market conditions, competitor activities, and other external factors influence campaign performance. Isolating the effect of these external influences from the campaign's incremental lift is challenging. - Some things take time
A marketing campaign's impact may not be immediate. Some campaigns are designed for long-term engagement and branding, making their incremental value hard to measure in the short term. - Data quality
Accurate incrementality measurement relies on high-quality, accessible data.
However, with advanced statistical techniques like DiD and access to accurate data, businesses can overcome these challenges and gain valuable insights into their campaign performance.
Causal inference and difference in differences (DiD)
Causal inference plays a crucial role in accurately understanding incrementality within eCommerce. It involves determining the cause-and-effect relationship between a marketing campaign and business outcomes.
The 'Difference in Differences' (DiD) approach is one method to achieve this. This statistical technique compares the change in outcomes over time between a group that is exposed to the campaign (treatment group) and a group that is not (control group).
By examining the differential effect, DiD helps isolate and attribute changes in sales or revenue specifically to the campaign rather than to external factors or trends.
What questions can be answered with incrementality measurement?
Incrementality measurement can help businesses answer important questions such as:
- Did a specific campaign or offer drive additional sales and revenue?
- How much did the campaign contribute to overall business outcomes compared to other factors?
- What is the incremental lift caused by different channels and campaigns?
- Which customer segments were most influenced by the campaign, and how did their behavior change?
Testing for incrementality
Testing for incrementality helps to understand the true impact of your campaigns on your target audience. It also allows you to measure the effectiveness of campaigns with no directly trackable outcome (e.g., highlighting key benefits or creating urgency based on delivery deadlines).
It measures how much of an increase in performance, clicks, sales, and other metrics you’ve achieved from running a campaign.
We can also assign a confidence value to the result, helping you make an informed decision about what action to take based on the results.

What is control group reporting?
Control group reporting is a way to measure how much an offer or campaign improves performance.
It does this by comparing people who were exposed to the offer or campaign (the treatment group) with people who were not (the control group).
If the results change for the better, we know the offer or campaign has a positive effect.

Validating control group reports
To ensure the analysis is robust with a good confidence level, we apply some statistical modeling:
- We take results from a date range where no changes were made to the campaign - this reduces noise from other factors affecting the result.
- We perform a Welch t-test on the uplift seen for the average order value, average items per order, and revenue per user to get a confidence score - this accounts for the different sample sizes.
- For conversion rate, we create a model of the rate based on Bayesian inference and a beta distribution for both the control and treatment groups and compare these using a concept of minimum uplift - this ensures the comparison is fair and accounts for the different sample sizes.
Example of a control group test
You want to find out if offering 10% off to new customers is driving more purchases. You could start this campaign and see if there is an uplift compared to the previous month, but there are many other reasons why an increase might happen:
- A new ad campaign has gone live
- Your brand is in the news
- A product you sell is trending online
- A change in the weather has made one of your products more appealing
You really want to know if the 10% offer is doing the hard work.
To do this, you would run a campaign for 10% off targeted at the new customer segment with a control group test added. We usually recommend a 90/10 split, but getting a statistically valid result depends on the traffic volumes and how long you run the test.
